Precision, Recall, and Their Importance for AI Detector Performance Evaluation
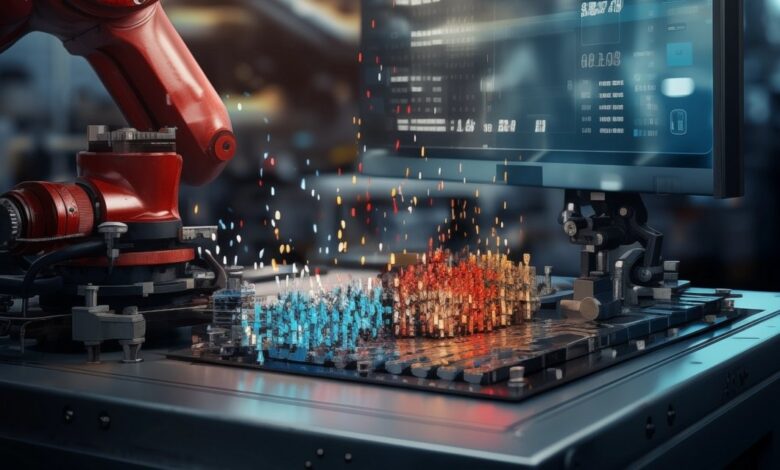
Detection and classification of AI content have become important nowadays in the rapidly changing environment of artificial intelligence. Such needs are highly imperative for applications in the domains of disinformation detection, copyright infringement, and deepfakes. Two major metrics include precision and recall. Although the notions sound quite technical, both of the measures play an important leading role in the real-world performance of a detector.
Understanding Precision and Recall
The precise definition of precision is the proportion of true positive items among the items the detector labeled as positive. In other words, it measures the accuracy of the detector in detecting true positive AI-generated content. High precision means that a detector does not confuse much human-generated content with AI-generated.
On the contrary, recall measures what proportion of actual positive items was the detector able to identify. It gauges the capacity of the detector to seize all things relevant to the AI-generated content. If the recall is high, that means how infrequently the detector will overlook aspects of AI-generated content.
While precision and recall are important technical metrics, let’s not forget that they are evaluated by humans. The role of an AI detector should not be only to perform accurate AI content identification but to support humans in making their decisions.
For instance, an AI detector is very precise but with low recall. That might mean the accuracy in identifying true AI-based content could be very high, even though it misses a lot of them, and thus in this aspect, the detector would be of lower effectiveness at protecting users from dangerous AI-generated content.
A high recall but low precision detector could detect a lot of AI content, but it would also misclassify human content as AI-generated, leading to false accusations, damage to reputation, and confusing situations that should not exist.
Precision-Recall Tradeoff
Often there is a tradeoff between precision and recall: Improving one metric often does so at the cost of the other. A detector that was designed to be most sensitive — that is, high in recall — to AI-generated content might also mistakenly identify human-generated content, leading to low precision. The opposite can also be true. A detector that is very cautious about labeling content as AI-generated — that is, high in precision — might miss some of the AI content, thus yielding low recall.
The trade-off between precision and recall is an application-dependent factor. For example, in a scenario where some false positives could lead to disaster, the importance would accrue in a high level of precision, such as in the case of accusing a person of plagiarism. On the other hand, a high recall might be more important in a case where all AI-generated content should be detected, even at the risk of some false positives.
Beyond Precision and Recall
While precision and recall are two of the most important measures applied in the evaluation regarding the performance of an AI detector, there is a lot more to it. Other relevant factors are as follows:
Efficiency: In terms of how quickly can the detector work through large amounts of data.
Scalability: In terms of how much can the detector take in — more data and more complexity.
Interpretability: In terms of whether the detector can be configured to explain its outputs.
HireQuotient AI Detector: Balancing Precision and Recall for Optimal Performance
HireQuotient’s AI Detector is designed to strike an optimal balance between precision and recall, ensuring that users can trust its accuracy while minimizing the risks associated with false positives or missed detections.
The HireQuotient AI Detector leverages advanced algorithms to achieve high precision, ensuring that AI-generated content is accurately identified without misclassifying human-generated content. At the same time, it maintains a high recall rate, capturing all relevant AI-generated content to ensure comprehensive detection.
What sets the HireQuotient AI Detector apart is its human-centric approach. The tool is designed not only to deliver technical accuracy but also to support users in making informed decisions. By providing clear explanations of its outputs and maintaining a balance between precision and recall, the HireQuotient AI Detector helps users navigate the complexities of AI content detection in real-world applications.
Conclusion
This brings precision and recall; for sure, they are powerful and telling means of gauging the performance of an AI detector, but not the alpha and omega. It is with an appreciation of the trade-offs in precision and recall among other relevant factors that we can improve and implement AI detectors accurately in major real-world applications.
The ultimate goal for these AI detectors would be to enlighten humans to make conscious decisions in an AI-ruled world that is progressively growing. We can thereby be assured that these detectors serve their purpose by looking carefully at how they perform.